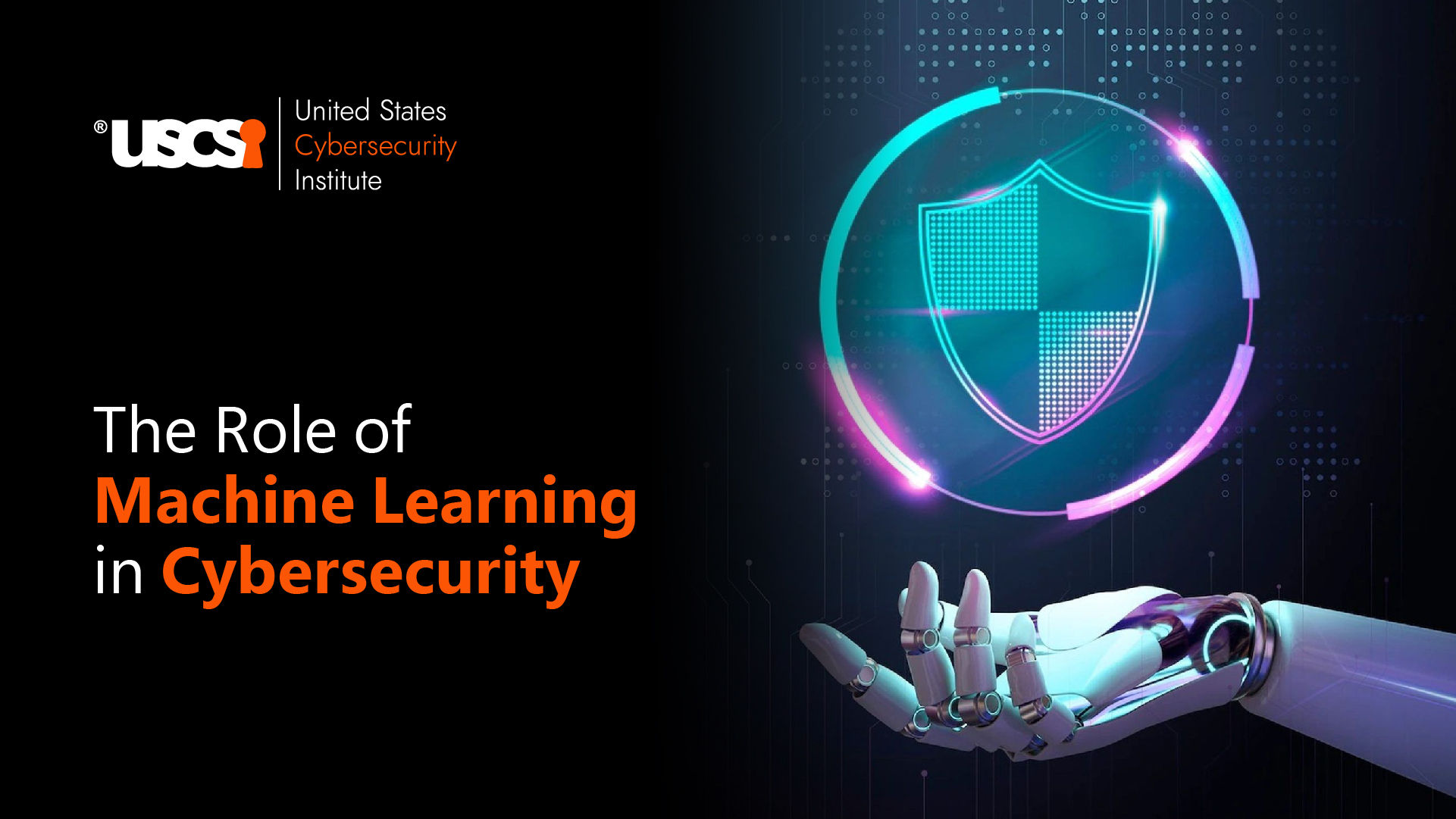
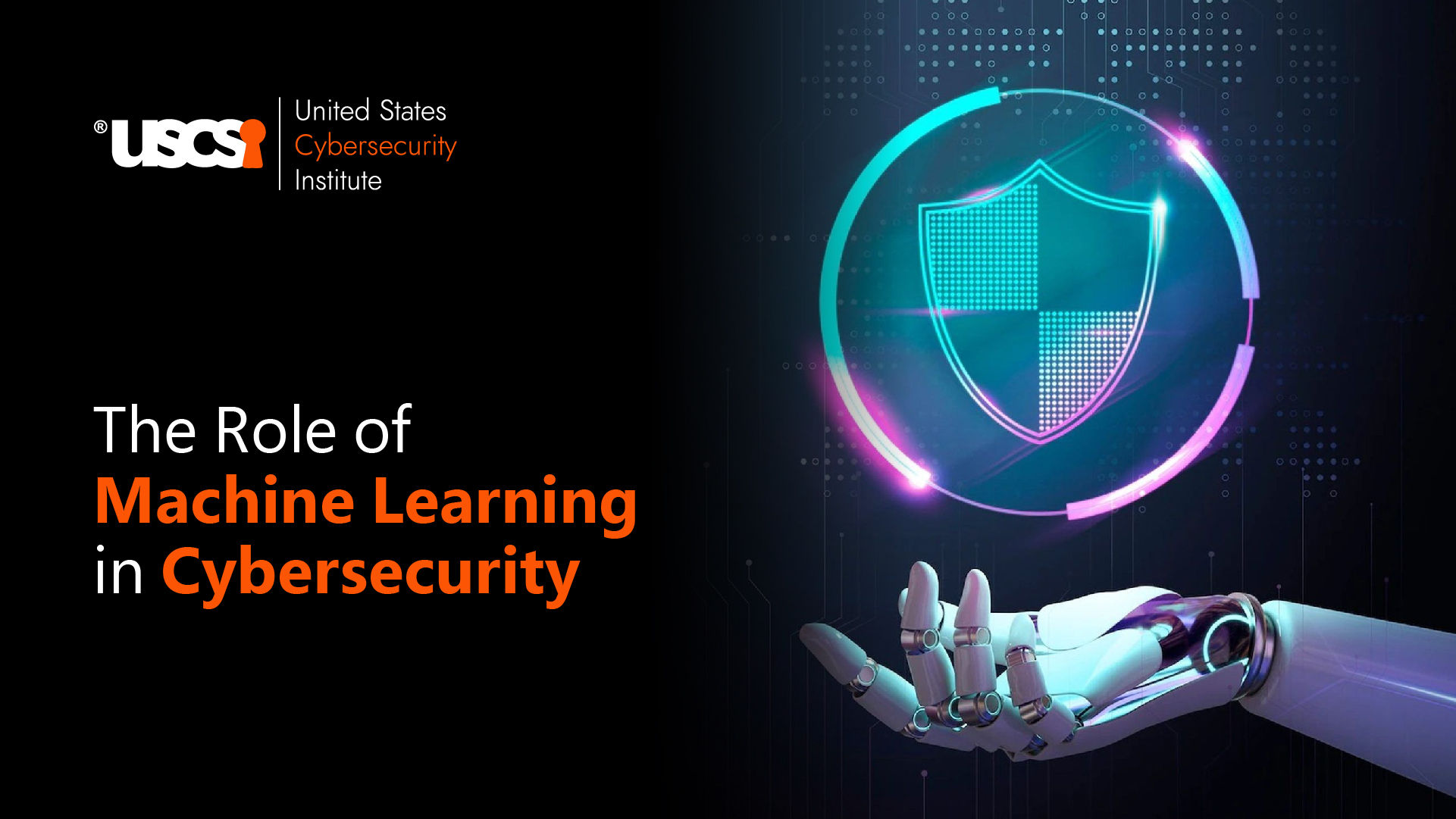
The Role of Machine Learning in Cybersecurity for Modern Businesses
The convergence of advanced technologies has the potential to revolutionize operations across several industries. One such convergence is artificial intelligence or machine learning and cybersecurity.
As the number of cybercrimes is constantly increasing and the cost of cybercrimes is growing at an astounding rate, relying only on traditional methods to protect an organization’s systems, networks, and data is not enough. Therefore, they must leverage advanced machine learning technology to strengthen their security measures and protect their digital assets and sensitive data from ever-evolving cyber threats.
Let us explore in depth the role of machine learning in cybersecurity and understand various ways in which it is used to enhance cybersecurity measures and strengthen security.
Growing Role of Machine Learning in Cybersecurity
Machine learning is a subset of artificial intelligence technology and machine learning models can learn from their experience on their own without explicit programming. The relationship between cybersecurity and machine learning is rapidly growing as organizations realize the importance of using such advanced technologies to strengthen security.
According to Statista, the AI in the cybersecurity market is expected to grow by roughly 134 billion U.S. dollars by 2030. This huge growth can be directly attributed to several powerful functions that machine learning can offer in cybersecurity such as:
- Predict and prevent attacks by identifying suspicious patterns
- Help with faster response and minimize the impact of cyber attack
- Detect unusual behavior to detect anomalies and isolate affected systems automatically
- Automate various security tasks
These benefits cannot be availed when using traditional rule-based or signature-based security solutions.
How does Machine Learning Work in Cybersecurity?
Using machine learning in cybersecurity is a complex process. However, using smartly, advanced machine learning models can significantly boost an organization’s cybersecurity efforts. Here is a basic process of how machine learning can be employed at various stages of cybersecurity and built and work:
- Collect high-quality data from various sources such as traffic logs, endpoint devices, threat intelligence feeds, etc.
- Processing data to eliminate incorrect and missing values, and detect outliers. Cleaning and standardizing it to utilize in machine learning algorithms.
- Identifying and extracting relevant features from the data that can be used to train the model
- Selecting the right machine learning algorithms as per specific requirements and use case
- Training the selected machine learning model on the prepared data so that they can learn the patterns and anomalies
- The models are then deployed into a production environment where they detect and monitor the potential threats in real-time
- They learn to use the insights and assist in decision-making. They also trigger appropriate security responses based on their intelligence.
Cybersecurity professionals must ensure they monitor the performance of the models continuously. Even though the model can continuously learn and adapt to evolving and emerging threats on their own, regularly updating and retraining models should be a mandatory part of their cybersecurity strategies.
Real-world applications of ML
Machine learning has a wide range of applications in the field of cybersecurity. Here are some of the notable ones:
-
Intrusion Detection Systems (IDS)
The IDS systems can use machine learning to analyze network traffic and identify unusual patterns that indicate malicious activities and potential threats. ML-powered systems can learn normal behavior and flag anomalies such as unauthorized access attempts or data breaches.
-
Malware Detection
Using machine learning algorithms, systems can analyze software behavior and easily detect malicious codes. They can examine file signatures, network traffic, system interactions, and other elements of software to identify and block malware even before they can cause much damage.
-
Machine learning can effectively detect anomalies in network traffic such as DDoS attacks or port scans. They can analyze network flows to identify and mitigate potential threats.
-
User Behavior Analytics (UBA)
It becomes easy with machine learning in cybersecurity to detect unusual activity by analyzing a user’s behavior patterns. This helps detect insider threats or compromised accounts. UBA systems work by establishing a baseline (standard) behavior and flagging or sending security alerts if there are any deviations from that standard.
-
Vulnerability Assessment
Machine learning models can be used to identify vulnerabilities (if any) in software and systems. They can analyze code and system configurations to predict weaknesses and suggest appropriate patching strategies on priority to security teams.
Limitations of Using Machine Learning in Cybersecurity
Cybersecurity and machine learning combined offer robust security to organizations. However, there are certain limitations or challenges of using machine learning in cybersecurity that need to be addressed.
First of all, a huge amount of data is required to train the ML models. High-quality and clean data aren’t always readily accessible and are not feasible to obtain.
On top of that, advanced machine learning algorithms lack the adaptability that human intuition offers and therefore, they can struggle with new and emerging threats. False positives and false negatives are other considerations that can lead to security lapses when not heeded properly.
Conclusion
Cybersecurity is a vast domain and consists of a lot of tasks to ensure the organization’s digital assets including sensitive data are protected from all kinds of cyber threats. Using machine learning in their cybersecurity measures, organizations can achieve a high level of efficiency, accurate responses, and faster action against attacks. However, they must effectively address the challenges associated with it. As we look into the future, we can see even more advancements in machine learning technology and a better convergence between cybersecurity, ML, and AI.